
Synthetic data will shape the future of AI.
Riding the Synthwave
There’s trouble in AI paradise, and this time it’s not Elon Musk or Sam Altman at the center. Rather, it’s an issue that threatens to upend any utopian (or dystopian) dreams of achieving Artificial General Intelligence (AGI) in the next half decade. That issue is data, or, the lack of it.
During my time as a solution consultant in B2B SaaS, I must have said the phrase “data is the new oil” no less than a thousand times. That phrase triggers a somewhat violent bout of nausea every time I hear it. It seems, though, that there is an ironic truth to it – in that we seem to be running out of this resource. A recent Statista chart shows that in 2024 humanity will generate about 147 zettabytes (that’s a 1 with 21 zeros after it) of data. Nevertheless, a recent Bloomberg article highlighted that Google, OpenAI, and Anthropic were struggling to get their AI to meet their new performance benchmarks. All three market leaders cited a lack of data as one of the primary causes of this struggle, the other being energy constraints in the US.
In a recent talk in the Harvard Kennedy School Institute of Politics, former Google CEO Eric Schmidt explained that the lack of publicly available data led to a consolidation of product features across competitors, pointing out the similarities between the major LLM models – ChatGPT, Claude and Gemini. All three have ambitions to be truly multimodal models, meaning that they can be fed any kind of input (images, text, video, etc.) and produce outputs that are as varied as the inputs. Yet, it seems that all three converged on a chat interface for a very sophisticated digital assistant, with only minor differentiation between them. Schmidt argues this is because these models sucked up all of the same publicly available data. AI giants and startups alike are feeling the heat to be better. Pressure to accelerate the development of AGI would create an undeniable geopolitical and technological advantage in a world on the cusp of transformation.
Enter synthetic data: the extra bit of oomph the innovation engine needs to cross the AGI finish line.
How Synthetic Data Works
Let’s start with a simple use case to understand what synthetic data generation is. Take the RC Marketing case, Artea. During the class discussion, we found that the online clothing retailer had uncovered algorithmic bias in the data model it used to generate its analytics insights. It seemed the company’s marketing material favored one racial group at the expense of others, and the question remained: how do they get rid of this bias within their data?
Synthetic data would let Artea take its real, historical customer data and supplement it with artificially generated user data that could better represent minority shoppers. In turn, Artea would be able to make better marketing decisions, knowing that it has not ostracized a group with its marketing tactics. Through synthetic data, we can look forward to more equitable representation in data used to train artificial intelligence models and minimize algorithmic bias in marketing.
The beauty of this type of data is that it allows us to circumvent many issues facing businesses working with real data today without breaching regulations. It allows us to create truly private and anonymized data in fields where data is sensitive and paramount, such as mimicking real patient data in healthcare or using artificial transaction data to train fraud detection algorithms in the financial payments space. It also allows us to fill in gaps in datasets where some data may have been lost in the day-to-day flow of work or due to accidental deletion. From a business perspective, generating synthetic data can potentially be much cheaper than collecting data “the old fashioned way” or purchasing it through data brokers.
That’s not to say that synthetic data is without its failings. The old computer science saying “garbage in, garbage out” rings true here. Synthetic data itself tends to be based on patterns found in real data. This means that if the data is low quality, incomplete or biased, then we would have effectively achieved nothing. Another potential issue could be in what is called noise generation. Humans are a highly heterogeneous bunch and so any dataset is bound to have some anomalies or blips that represent the irregularity of the human condition. Sometimes, synthetic data produces datasets that are too “smooth” and lack the irregularity and occasional outright strangeness of truly random human data. Therefore, a good synthetic data pipeline would add some degree of noise to the data to approximate randomness and outlying data points.
If you’re thinking that this all sounds very AI expert and Data Science-y, you’re not entirely wrong. There’s no need to hang out in the valley of despair, however, as a bunch of synthetic data companies are helping businesses both generate and integrate this kind of data into their workflows.
The Nascent Industry
There are about fifty-eight businesses of various sizes operating within the space. Competitors tend to focus on either structured data – data found in tables or databases – or unstructured data, which doesn’t have a fixed format. Those of us who listened in on LEAD in RC year would recognize Coohom (of protagonist “Starburst” fame) as one of the players in the unstructured data space. Regardless of data type, most companies would follow the same machine learning workflow as in the chart below.
Most lifecycles begin with a small real data gathering exercise, followed by a bit of data prep and cleaning. Once this data is gathered, we can then generate synthetic data based on it and mix it into our training data set. We would then proceed with our usual machine learning model training, with synthetic data providing its built in advantages of preserving privacy and adding explainability to the data, since we know exactly what it is made of and where it comes from.
The good news there is that many of these platforms have put in a lot of work to make their services accessible to the AI-dabbler as well. Platforms such as Mostly.ai provide a seamless UI with built in AI assistants to help users navigate the lifecycle without requiring an engineering degree from MIT. The even better news is that a good chunk of platforms have demos and free trials to allow people to get up and running with their platforms, along with documentation designed to cater to different types of users from less technical to more technical.
So, if you’re a future founder, joiner, or business leader, keep an eye out for synthetic data. Regardless of whether you’re an AI optimist or pessimist, forecasts show that by 2030 most of the data that will be used to train AI will be artificially generated. Some of us attending HBS today may one day help shape the future of AI. Let’s use tools like synthetic data to make sure it’s a good one.
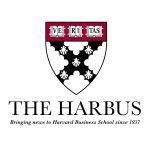
Youssef Abouelnour (MBA ’26) comes from Cairo, Egypt. Prior to HBS, Youssef spent 7 years working as a Sales Engineer for SAP covering emerging tech. He is currently on the hunt for the best hot chocolate in town and looks forward to seeing snow for the first time.
Commentaires